News / Tech News |
AI makes retinal imaging 100 times faster, compared to manual method
Researchers at the National Institutes of Health applied artificial intelligence (AI) to a technique that produces high-resolution images of cells in the eye. They report that with AI, imaging is 100 times faster and improves image contrast 3.5-fold. The advance, they say, will provide researchers with a better tool to evaluate age-related macular degeneration (AMD) and other retinal diseases.
A top-down view of lab-grown RPE cells as seen with high-resolution microscopy. Unlike AO-OCT, which is performed in an awake person, this image was created with preserved tissue. Photo: NEI
“Artificial intelligence helps overcome a key limitation of imaging cells in the retina, which is time,” said Johnny Tam, Ph.D., who leads the Clinical and Translational Imaging Section at NIH's National Eye Institute.
Tam is developing a technology called adaptive optics (AO) to improve imaging devices based on optical coherence tomography (OCT). Like ultrasound, OCT is noninvasive, quick, painless, and standard equipment in most eye clinics.
While adding AO to OCT provides a much better view of cells, processing AO-OCT images after they’ve been captured takes much longer than OCT without AO.
Tam’s latest work targets the retinal pigment epithelium (RPE), a layer of tissue behind the light-sensing retina that supports the metabolically active retinal neurons, including the photoreceptors.
The retina lines the back of the eye and captures, processes, and converts the light that enters the front of the eye into signals that it then transmits through the optic nerve to the brain. Scientists are interested in the RPE because many diseases of the retina occur when the RPE breaks down.
Imaging RPE cells with AO-OCT comes with new challenges, including a phenomenon called speckle. Speckle interferes with AO-OCT the way clouds interfere with aerial photography.
At any given moment, parts of the image may be obscured. Managing speckle is somewhat similar to managing cloud cover.
Researchers repeatedly image cells over a long period of time. As time passes, the speckle shifts, which allows different parts of the cells to become visible. The scientists then undertake the laborious and time-consuming task of piecing together many images to create an image of the RPE cells that's speckle-free.
Tam and his team developed a novel AI-based method called parallel discriminator generative adverbial network (P-GAN)—a deep learning algorithm.
By feeding the P-GAN network nearly 6,000 manually analyzed AO-OCT-acquired images of human RPE, each paired with its corresponding speckled original, the team trained the network to identify and recover speckle-obscured cellular features.
When tested on new images, P-GAN successfully de-speckled the RPE images, recovering cellular details. With one image capture, it generated results comparable to the manual method, which required the acquisition and averaging of 120 images.
With a variety of objective performance metrics that assess things like cell shape and structure, P-GAN outperformed other AI techniques.
Vineeta Das, Ph.D., a postdoctoral fellow in the Clinical and Translational Imaging Section at NEI, estimates that P-GAN reduced imaging acquisition and processing time by about 100-fold. P-GAN also yielded greater contrast, about 3.5 greater than before.
By integrating AI with AO-OCT, Tam believes that a major obstacle for routine clinical imaging using AO-OCT has been overcome, especially for diseases that affect the RPE, which has traditionally been difficult to image.
“Our results suggest that AI can fundamentally change how images are captured,” said Tam. “Our P-GAN artificial intelligence will make AO imaging more accessible for routine clinical applications and for studies aimed at understanding the structure, function, and pathophysiology of blinding retinal diseases. Thinking about AI as a part of the overall imaging system, as opposed to a tool that is only applied after images have been captured, is a paradigm shift for the field of AI.”
YOU MAY ALSO LIKE





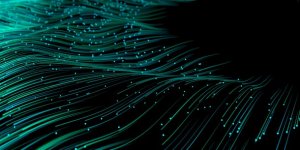

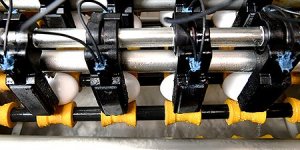




